The Ultimate Guide to Clause Libraries
A step-by-step guide
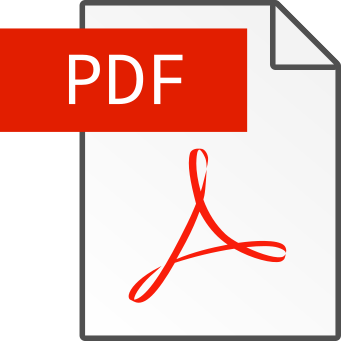
Legal professionals are busy people. They often cite time commitment as their main impediment to building a clause library – not just the time to add the clauses but also to maintain and update them. The question typically goes “Can artificial intelligence (AI) not build this for me?”
For the sake of simplicity, we label all software tools that automatically build clause libraries as “AI-based”, because that’s the terminology used by most marketing departments. There are actually quite some differences between “AI”, “data mining”, “supervised learning” and “unsupervised learning”, but we are trying to keep it 101.
Indeed, a number of AI-powered tools (Draftwise, Genie AI’s SuperDrafter, Henchman) have popped up recently that allow you to extract vast amounts of clauses from the documents your organisation has lying around.
So, is this technology up to snuff?
AI-based clause library software tools do a good job in letting you search for a clause using keywords. Such keyword searches are mature and widely used in every industry. Accordingly, you can be confident that when you are searching for “employee liability”, you will indeed get a list of clauses that contain those words. Some of the more advanced tools will even automatically “cluster” clauses on the basis of the words in the clauses, so that similar clauses can be automatically associated with each other.
What’s really attractive about these tools, is of course that they require no time investment from legal experts. Your IT department will install the tool, and you are immediately good to go. If all you need is to occasionally find that one clause you had written in the past, this seems like the perfect solution.
So what’s the catch?
Finding unique keywords... Keyword-based searching works great with unique keywords (“bankruptcy”, “force majeure”) or specific expressions (“material adverse effect”). Unfortunately, these are a minority, because many clauses tend to be made up of a relatively small number of unique keywords. For example: words like “party”, “confidential”, “material”, “liable”, “obligation” are repeated in many different types of clauses.
In many clauses, the most interesting keywords will often be missing. For example, when a corporate lawyer wants to insert a “Texas shootout” clause, a useful keyword might be “shootout”. Unfortunately, that word is most likely not literally present in the clause text itself, so an AI-tool will be at a loss to find a clause like that using a keyword search. Similarly, a commercial lawyer will frequently want to find a clause with a low liability cap or a maximal responsibility carveout, but real-life clauses don’t literally mention such qualifications for obvious reasons. As a result, you will have a hard time finding such a clause, because it will typically be made up of a series of popular keywords (“share”, “buy”, “sell”, “price”, “liable”, and so on).
You need to feed the software gigabytes of data to make it somewhat smart. As a rule of thumb, you need to have at least several thousand different versions of each relevant clause in your jurisdiction to allow the software to completely independently figure out that two pieces of text are actually variations of a same clause (near-identical copy/pastes don’t count). Even the largest UK/US law firms would perhaps only come close to this amount when including material received from clients and counterparties, or when including intermediate versions during negotiations — but it’s probably not wise to randomly include such material.
When the volume of data is not sufficient, you will not get Google-like search satisfaction. Instead, you will have to dig through long lists of chaotic, half-baked results.
Law is a profession of words, and drafters are all experts in massaging text. As a result, it can take a minute (particularly with long clauses) to interpret a clause and determine what it is really saying and, and what is deliberately hidden. If all you get back from your software consists of bare clauses, you will spend a lot of mental processing time understanding each search result. Over time, across a team, all this time spent interpreting search results quickly adds up — undermining the efficiency you were pursuing.
When clause libraries are automatically constructed, quite some confidential information will inadvertently get embedded into the database. As a result, everyone who uses the library will see confidential bits & pieces flying around when scrolling through the search results. This can easily breach typical confidentiality obligations in NDAs (“access must be restricted on a need-to-know basis”) or local bar rules.
When you mass-feed old files to your software tool — particularly when hosted by a third party — you are reusing documents containing often highly sensitive personal data for a purpose (your drafting comfort) that is incompatible with the initial purpose (handling a client file). With EU authorities increasingly upholding strict interpretations of the GDPR, such “repurposing” of personal data could be considered a fundamental breach of the Regulation. While automatic “scrubbing” will remove some personal data, it will never reach the extremely high anonymisation level that data protection authorities require.
To give the lawyerly answer: “it depends”.
If you are a solo lawyer and mainly want to search through your old clauses, they can be a good fit. You know your own material well, so you know which keywords to use. And you can probably quickly reconstruct the context that is missing in each search result. Confidentiality issues should be minimal, and if you work outside the EU you likely won’t be impacted by the GDPR.
If you are part of a legal team, the assessment becomes different, because you will have to balance the advantage of near-zero upfront legal expert time with the downside of spending relatively more time scrolling through — and mentally interpreting — the search results. You will also have to consider your risk position with regards to potential compliance issues.
In the end, the assessment probably boils down to how you look at a clause library.
Several hours or days
Negligible
Negligible
Distributed over time
High
High
Low
High
High
Low
Missing
Augmented clauses
High
Low
If you want to really understand the technical side of how AI plays a role in these situations, see the breakdown below. It’s not necessary to help you build clause libraries, but we’d hate to deny anyone the opportunity to geek out a little.
AI has made enormous strides over the past few years, from GPT-3 writing articles to Tesla’s self-driving cars. Even more established technology like machine translation or online search engines like Google have seen their functionality vastly improve in recent years.
The number one factor of success for all of these AI-powered technologies has been the sheer volume of data that has been made available to help train the AI. GPT-3 had to parse over 45 terabytes worth of text — that’s about 30 billion pages — with a capacity of about 175 billion machine learning parameters to get to where it is now. Tesla had to subject its AI to billions of miles of driving before it could reach a level of technical viability on highways. Similarly, machine translation engines incorporate millions of language pairs from which they distil their knowledge (e.g., all the statutory provisions translated by EU parliament translators).
If huge amounts of data are available, such "narrow” applications of AI are like magic, as anyone will have to admit when using modern translation engines such as DeepL, when cruising on the highway with Tesla, or when realising that Google autocompletes your search query as if it can read your mind. However, none of these technologies actually understand what is going on: they merely calculate statistical correlations, but with sufficient data available such correlations feel like magic.
If you want a good example of how easily you can trick the AI of the most advanced search engine on the planet, try googling “restaurants near me that are not McDonalds”. You will be surprised to see that almost all restaurants listed will be… McDonalds.
Similarly, even advanced translation engines have difficulties understanding context. In the example below, any legal expert will understand what “boiler plate” means. The translation engine translates it to “plaque chauffante”, i.e. a heating plate…
Besides the required volume, there is a second hurdle to overcome: comprehension. Even software that claims to be “self-learning” is not doing any actual "learning”, in the sense of how human beings would acquire new skills. The software will merely look for patterns that have statistical significance. And even with large amounts of data, this can go completely wrong.
Take the story of the AI that was trained to distinguish between wolves and huskies.
The software learned to identify them successfully, achieving very good accuracy with the sample images fed to it. But when practiced on other photographs, the software completely failed. The reason? From the software’s perspective, the different between a wolf and husky was the presence or omission of snow in the background. When confronted with a picture of a wolf in a snowy landscape, the software immediately assumed that it was looking at a husky…
Applied to the legal sphere: let’s say you are trying to teach AI to recognize a governing law clause. You can feed it millions of examples, but the exact lessons it will pull from that exercise are anyone’s guess. If a few too many of these governing law clauses refer to “Delaware” as the applicable law, it might assume that governing law clauses are all clauses that contain the word “Delaware”.
When it comes across a party introduction clause including a legal entity incorporated in Delaware, it may flag it as a governing law clause. When it comes across a clause selecting “New York” as its governing law, it may not recognize this as being a governing law clause.
A final problem is that it can only learn from what is present in the examples given to it. This is particularly problematic for a clause library, because what is not written in a clause is probably as important — and sometimes even more important — than what is explicitly written down.
This can become a particular hurdle for AI in continental Europe, where statutory provisions (e.g., articles of the Civil Code) tend to function as “fallback” provisions that will apply even when clauses are silent about that topic. The typical example is good faith in contract law: in many European jurisdiction, parties do not need to mention it for parties to be obliged to act in good faith towards each other. From the AI’s perspective, there will be a significant difference between clauses that explicitly mention good faith, and those that don’t. From a continental lawyer’s perspective, it will depend on the context, the drafting style, the length, and so on whether a clause that explicitly mentions good faith is indeed different from the same clause that omits any reference to good faith.