WEBINAR: Generative AI in Legal – Are you missing the boat?
ClauseBase co-founders Maarten Truyens and Senne Mennes recently hosted a webinar providing a very long answer to the number one question lawyers have asked us these past few months: “Am I missing the boat”? Below is a transcript of a curated section of the webinar.
If you are more audio-visually inclined or want to see the whole thing, you can find the recording here.
***
In recent weeks, we've experienced a sudden surge of interest in our product offering, with numerous lawyers reaching out to us — some familiar faces we hadn't spoken to in years; others we hadn't encountered before.
The common thread in those chats boiled down to a single question:
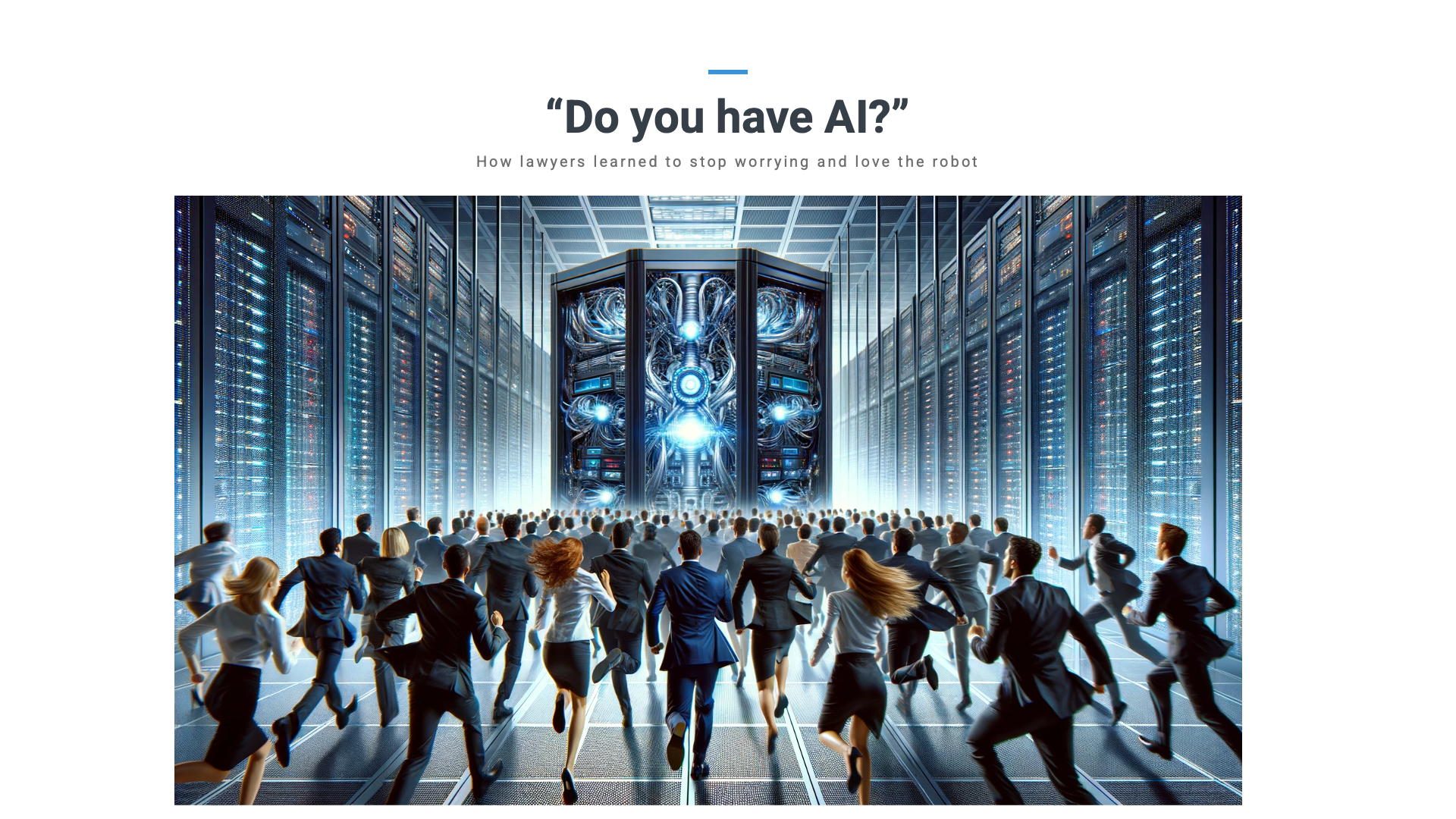
In past interactions with lawyers, we noticed a fear that AI might threaten their job. Interestingly,seems to be in the process of being replaced by a concern about lawyers utilizing AI. Who isn’t tired of the saying “AI won’t replace you; lawyers using AI will”, by now?
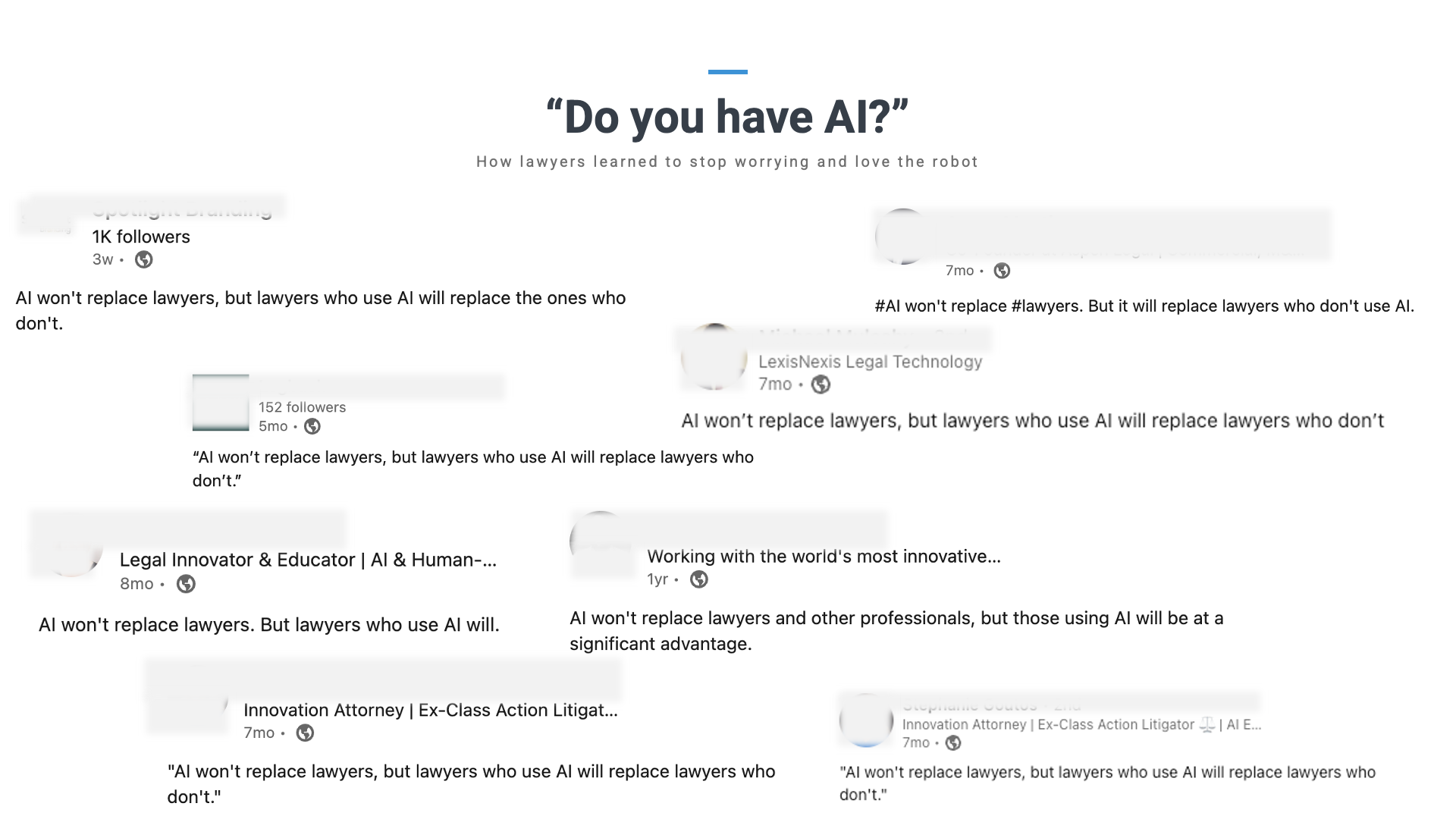
Another reason we are hosting this webinar is the sheer amount of disinformation in the market.

Taking advantage of lawyers' limited technological understanding and unfamiliarity with software procurement, there's an influx of unrealistic marketing and claims that simply cannot be met.
Our aim is to set the record straight.
Fundamentals of Generative AI
Our goal is to provide you with insights into what is and isn't possible with Generative AI.
There’s only one problem: the technology evolves so fast that whatever we share today will probably be completely outdated in a month or two.
That’s why we’re going to go over the fundamentals of LLMs in abstract terms: how they work and what their limitations are. That way, you have the necessary framework to evaluate GenAI as the technology grows and decide for yourself whether the information we are providing is still up to date.
Let's begin with the absolute basics: How do LLMs work?
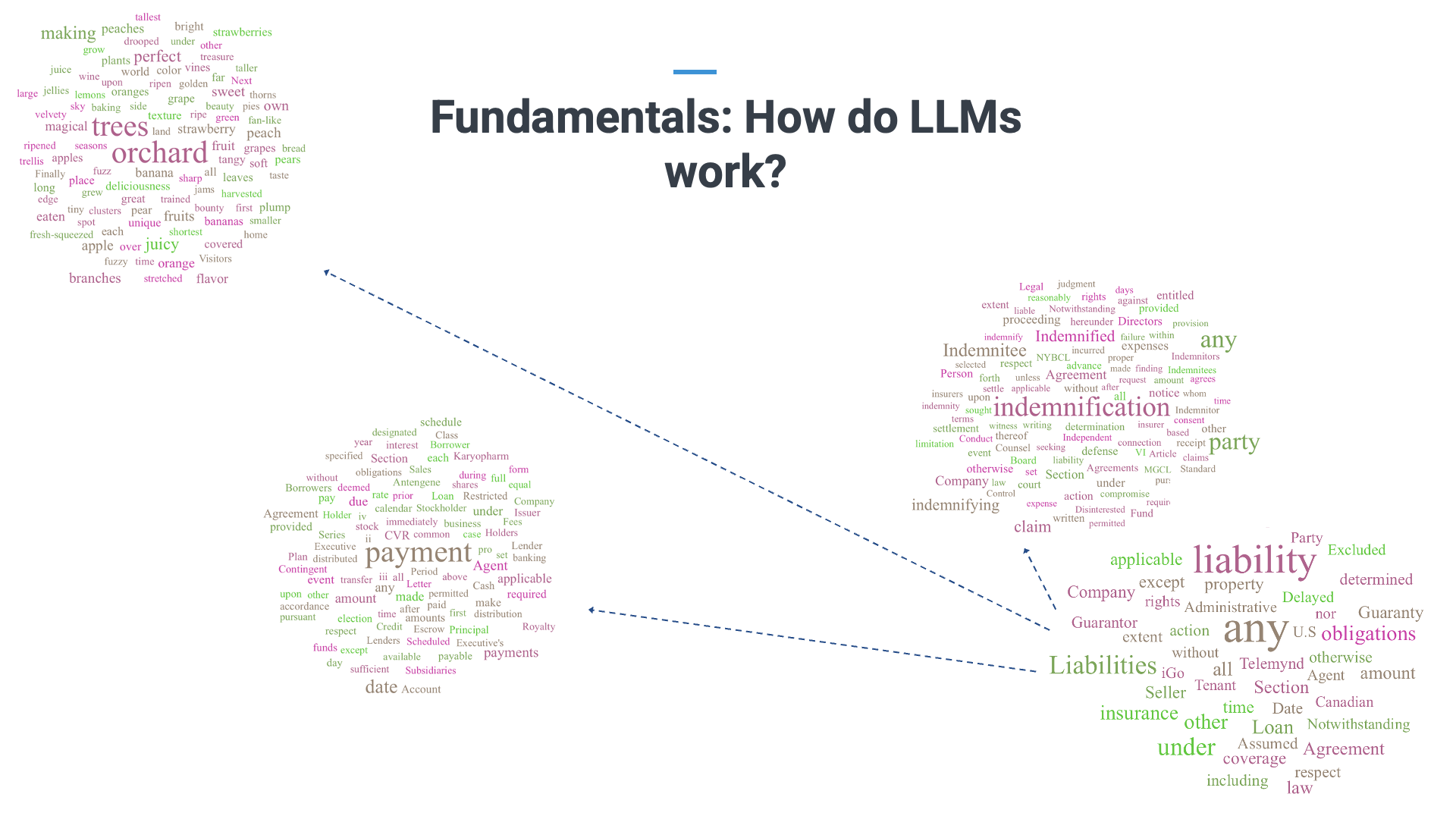
Essentially, they're trained to learn associations between billions of words, resembling the way our brains function with neurons firing in an unorganized manner. LLMs, like our brains, don't store hard facts in a specific location; instead, it's all about associations.
If you ask about a topic, the LLM reconstructs information from memory, much like recalling experiences. If you ask me to tell me about the holidays, I will reconstruct that experience from memory and maybe forget something, or fabricate something. But most importantly, it’s not like I open up a drawer somewhere in my memory bank. If you ask me now and ask me again in a day, you’re not going to get an identical response.
Thsat’s also how LLMs work.
LLMs don't retain conversation history; they immediately forget once they provide an answer. This human-like thinking power enables them to handle language creatively, yet it also leads to a significant challenge— "hallucination." LLMs, like humans, may misremember or fabricate information. Think of the numerous lawyers (e.g.: here and here) who have been caught using AI-generated content, citing case law that was entirely fabricated.
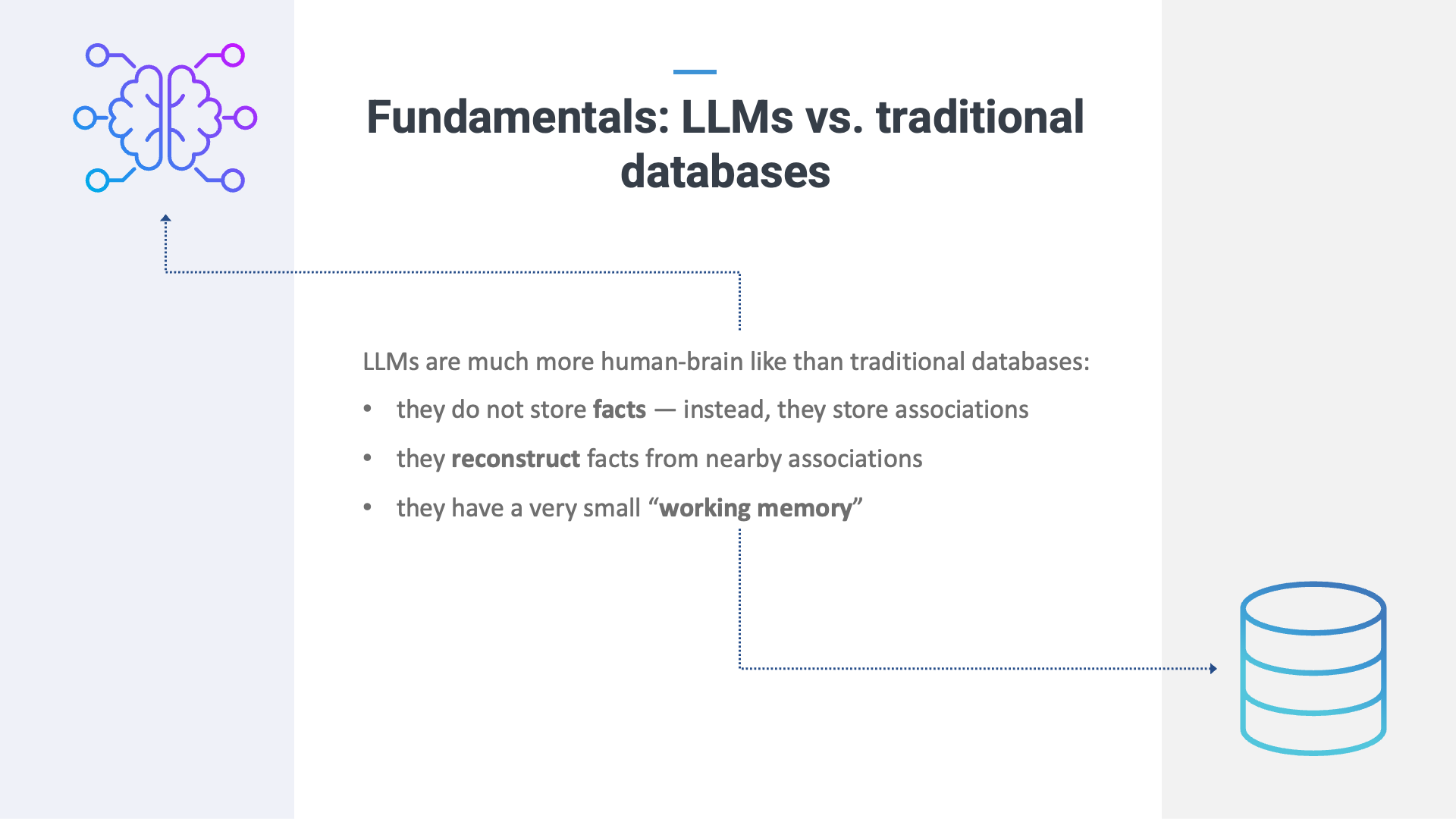
Another limitation is the "context window," similar to a human's attention span when reading. While LLMs can store vast amounts of background information, there's a limit to how much custom text they can consider when generating responses (which has to include the question posed by the user as well as the material on which they are asking the question, like a document or even an entire database). Currently, the battle among LLMs revolves around expanding this context window. At the time of writing, GPT4-Turbo holds the record at about 100,000 words.

But even if you stay within the limits of their context window, LLMs are not infallible. Research shows that once you submit a large amount of text, the LLM will start getting fatigue in the second half of the document. A bit like a human being reading a short paper of 5 pages versus a book of 200 pages: you cannot expect that all information will be remembered to the same degree.

Techniques like "Retrieval-Augmented Generation" (RAG) have been developed to combat the weaknesses of the limited context window. RAG acts as a filter applied before sending information to the LLM, breaking down large text chunks into manageable portions. However, this method has challenges of its own, it frequently happens that a chunk is erroneously labelled as "not relevant", thus leaving the LLM with insufficient information for an informed response.

Another approach to assist the LLM in dealing with large volumes of text correctly is "prompt engineering," where human experts curate legal information for the LLM. This manual effort improves results but is time-consuming. Additionally, LLMs are trained on broad datasets, not specifically for the legal sector. They struggle to distinguish between laws in different jurisdictions, highlighting the need for domain-specific training.

Finally, another problem is the available training data.
LMs are trained on a vast collection of very general data. Some legal data also managed to get included, but off-the-shelf LLMs were never trained specifically for the legal sector. As a result, they know what legalese feels like but have no real feeling with the material. For example: they have a very hard time distinguishing between different laws applicable in different jurisdictions.
Note that we say “off-the-shelf LLMs” here. It is possible that an LLM gets created that IS trained specifically for the legal sector, but the problem then becomes that all the good data to train this model on is either not available online (e.g.: case law that goes unreported) or hidden behind paywalls like legal research tools.
We’ve addressed this in further detail in a different webinar which you can find on our YouTube channel. Essentially, you can think of LLMs as a supercar that can get you to your destination much faster than any previous technology. However, a supercar can only go as fast as the road allows it to. Legal data is that road. The better data it has access to, the faster it can go. Having a supercar is great, unless all you have to drive it on is a bumpy country road…

What is not (yet) possible?
Let us now apply these limitations to concrete use cases. Perhaps the most interesting use case, and the most desired by many law firms we talk to, is what we’ve dubbed “LegalGPT”.
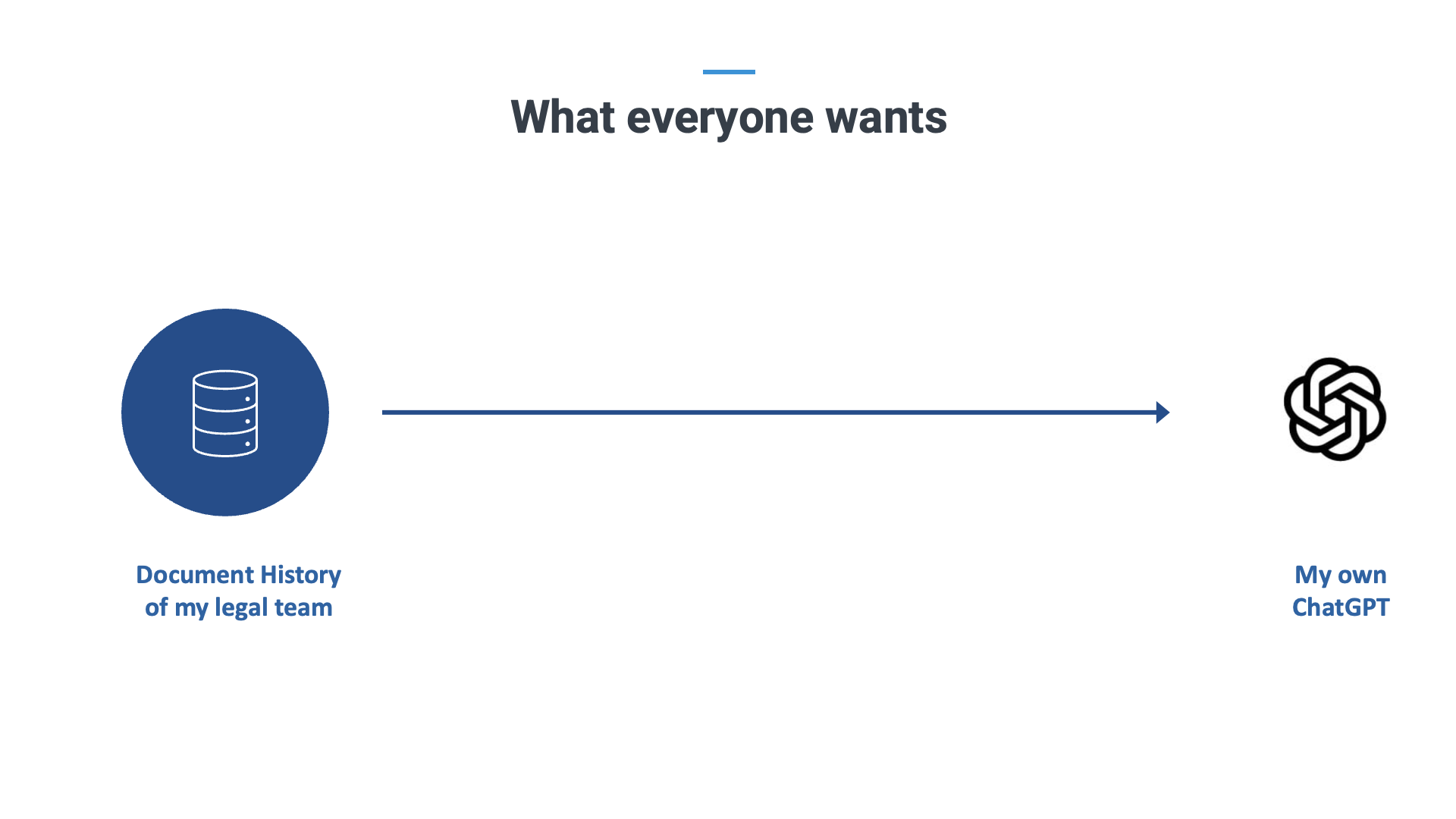
Unfortunately, everyone forgets about the intermediate step of training the LLM.
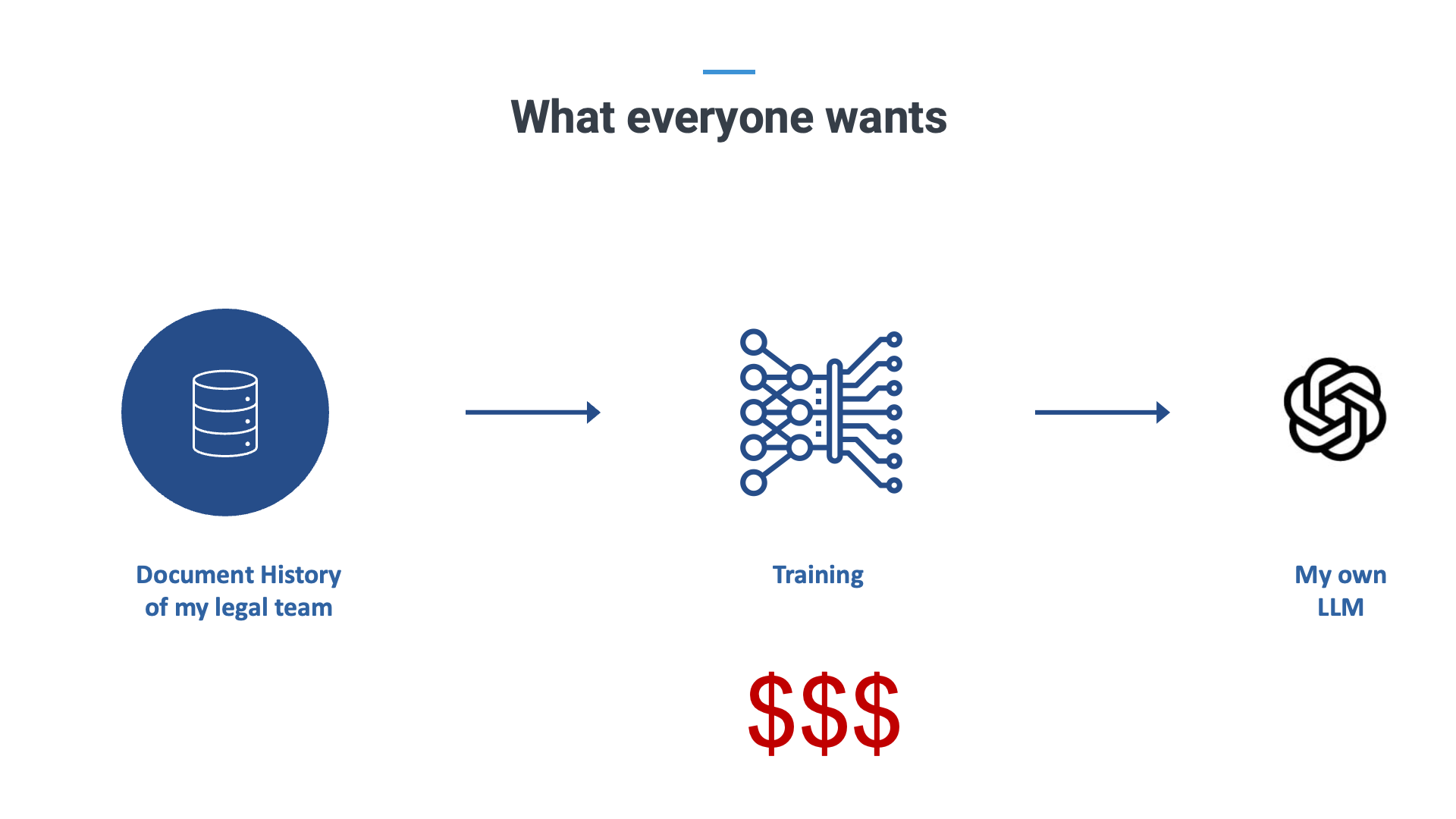
This requires terabytes of data, thousands of computers, millions of dollars, a huge team of experts, for every department. This exercise then needs to be renewed every year because the law always evolves. The teams that can make this kind of investment would really have a LegalGPT. The question only remains how many of those currently exist. We do want to emphasize that training costs for LLMs may go down in the future, but we’re not there yet.
The important bit to remember here, is that you cannot permanently “teach” an LLM something that is based on an analysis of thousands of documents at the same time. For this, you must train the LLM. And it is expensive.

What does work, is a combination of RAG and prompting. This combination doesn’t remove the limitations of the context window or the prefiltering mistakes of RAG, and you’ll still need to be able to offer clean data (and no, uploading your entire DMS does not count). But you will be leveraging LLMs where they excel – at the paragraph level, not at the database level.
Market outlook
Knowing what can be done and what cannot be done, let’s look at some practical applications of Generative AI that lawyers are already putting in practice.
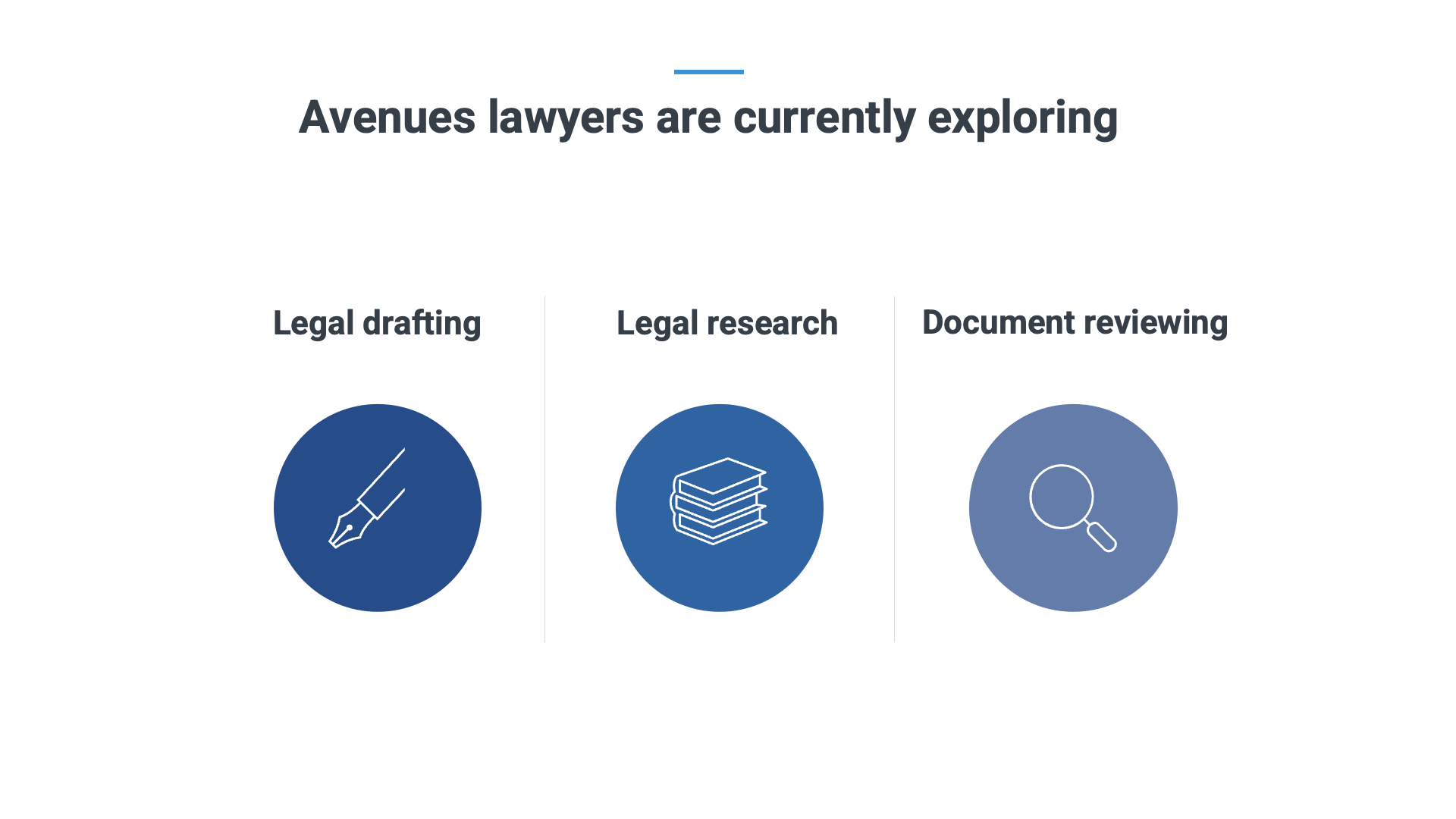
Legal Drafting
One of the most common uses of Generative AI is with a simple subscription to one of the off the shelf LLMs. Short prompts for emails or clauses such as "write a comprehensive confidentiality clause for a franchise agreement with a strict damages clause" are already being used by tons of lawyers in the field and can provide a useful starting point for the lawyer who is primarily looking for inspiration and does not immediately find anything in his or her firm's precedents. The only drawback is that hallucination may still occur in this situation. However, if you’re just looking for inspiration, this is not a major obstacle.
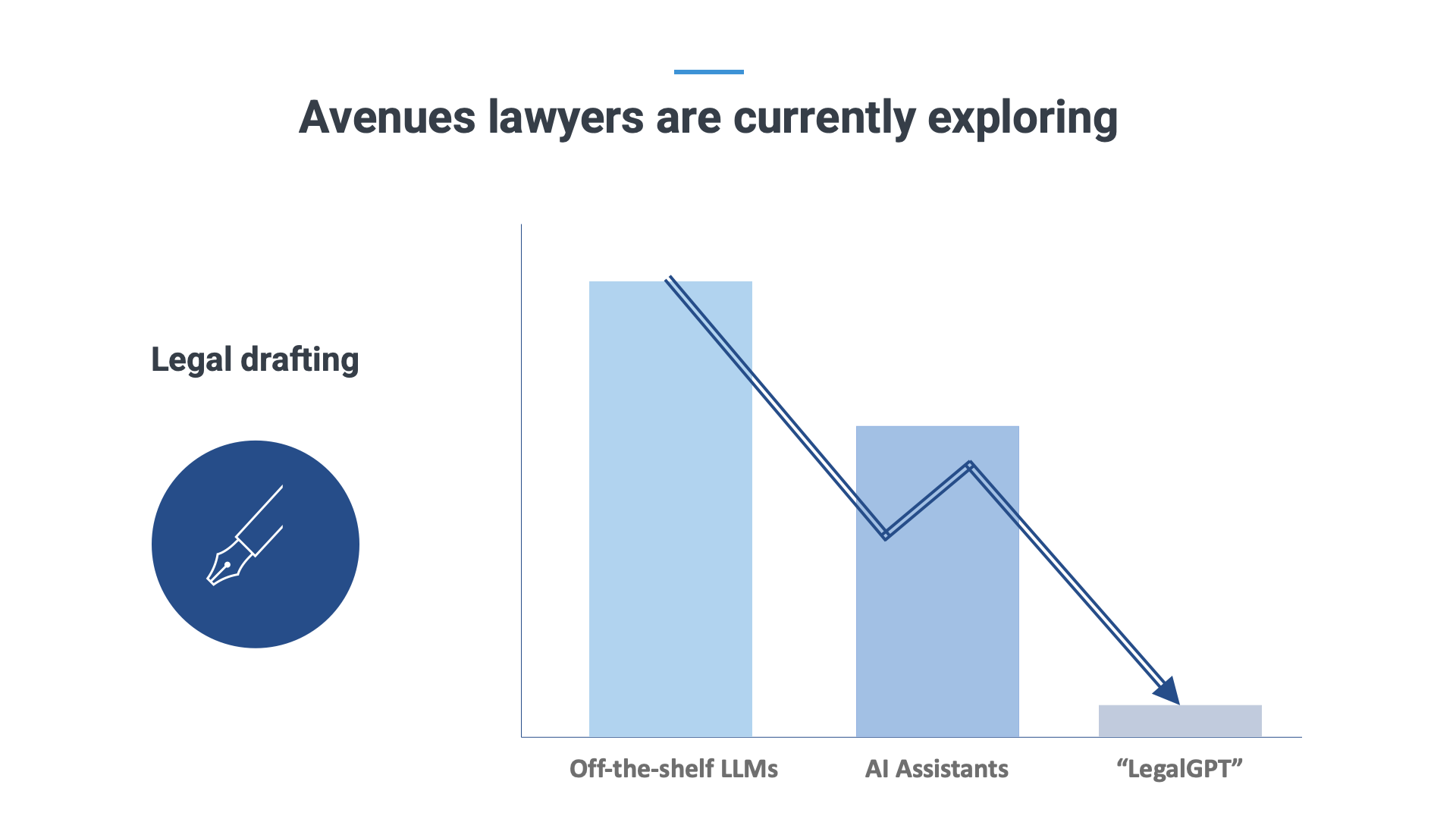
Obviously, there are caveats. In order to trust lawyers with basic, off-the-shelf LLMs for this kind of purpose, they need to be aware of the risk of hallucination (so you don’t use it for citing case law) and they need to make sure they are experts in the field of whatever they are requesting the AI’s aid with. If you’re not an expert, you can’t verify the output, which can lead to all kinds of trouble. It’s for those two reasons that some firms have decided to actually ban LLMs because they can’t guarantee that lawyers will abide by these rules.
On the other hand, many legal tech companies (yours truly included) have capitalised on the recent developments in Generative AI to launch Legal AI Assistants in different shapes and forms. These tools differentiate themselves from off the shelf LLMs by leveraging their functionalities for more legal-oriented tasks. This can include redrafting text in a Word document based on a short prompt, making the terminology across a document consistent, creating documents from scratch, and more.
Below are a few examples of how ClauseBase's own AI Assistant built into ClauseBuddy tackles some of these use cases:
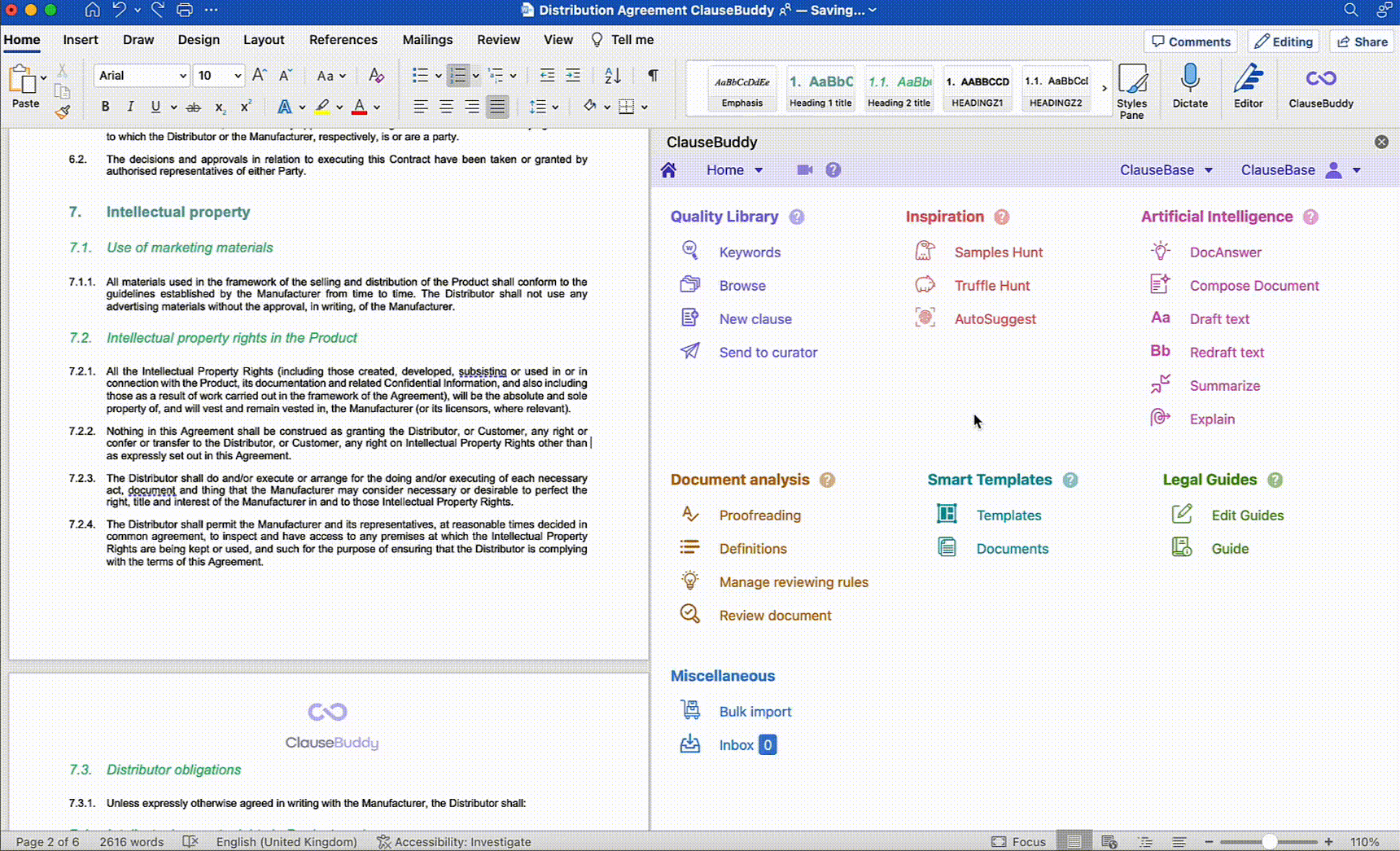


Document Reviewing
Reviewing an individual document is also a doable task for the modern generation of LLMs. Leaving confidentiality concerns aside for a moment, it is perfectly possible, for example, to upload a counterparty’s contract to tools like GPT-4 and quiz them on that document.
Our own Legal AI Assistant ClauseBuddy allows you to do that for any document you have opened in Word.
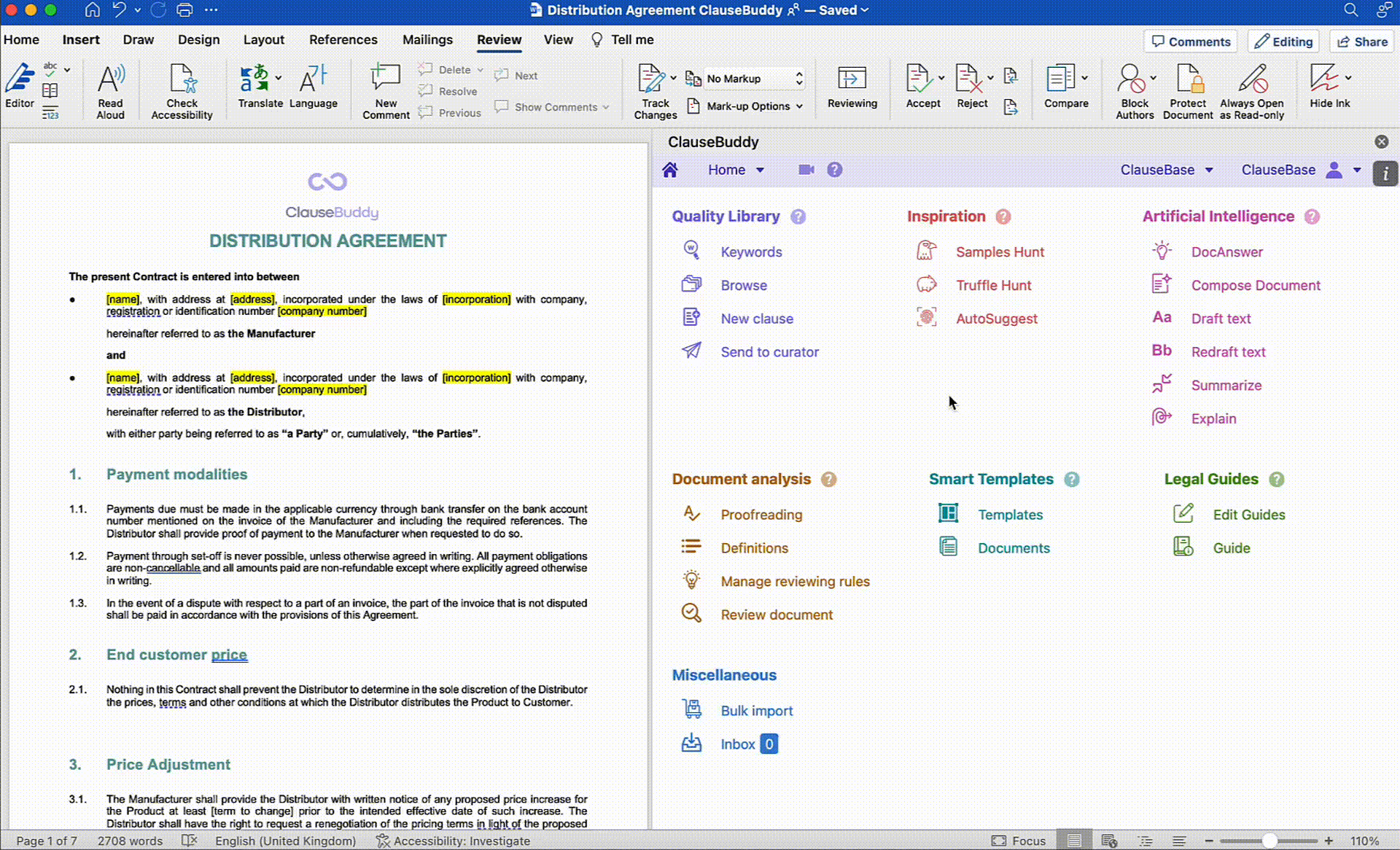
Questions such as "what is the applicable law?" and "what obligations does my client have in terms of payment?" will usually be answered correctly. This is again because such questions do not exceed the limits of the context window, the answers are usually localised (not spread across clauses) and we deploy them the LLM on what it is good at - semantic analysis of text.
Today, lawyers are also using this technology in conjunction with their contracting policies (which include things like what negotiation positions are or are not allowed, what fallback clauses are available, etc.).

This policy is then uploaded to the LLM and the LLM can then fully automatically perform the necessary checks to see where the contract is in line with the policy and where it is not.
Legal Research
Finally, Generative AI offers very many opportunities in the field of legal research.
Today, lawyers still spend an extraordinary amount of time ploughing through databases full of legislation, legal doctrine, and case law to answer legal questions.
Many suppliers of these databases are already experimenting with integrating their offerings with the more powerful LLMs, with Thomson Reuters recently announcing a series of such integrations with existing products such as Westlaw and Practical Law. LexisNexis similarly launched Lexis+ several months ago.
Again we have to emphasize: LLMs are particularly strong in semantic analysis. They can use RAG to plough through these large volumes of text and bring up useful passages from different, diverse sources. This can be a useful alternative to the individual searches for literal search terms that lawyers have to do in the traditional way and can ensure that they find sources they would otherwise have missed. However, what they cannot do is to analyse these principles and build their argument around the conclusion they draw in a legally correct manner.
Are you missing the boat?
Which brings us to the crux of this presentation and the question we started things off with: “Are you missing the boat?”…
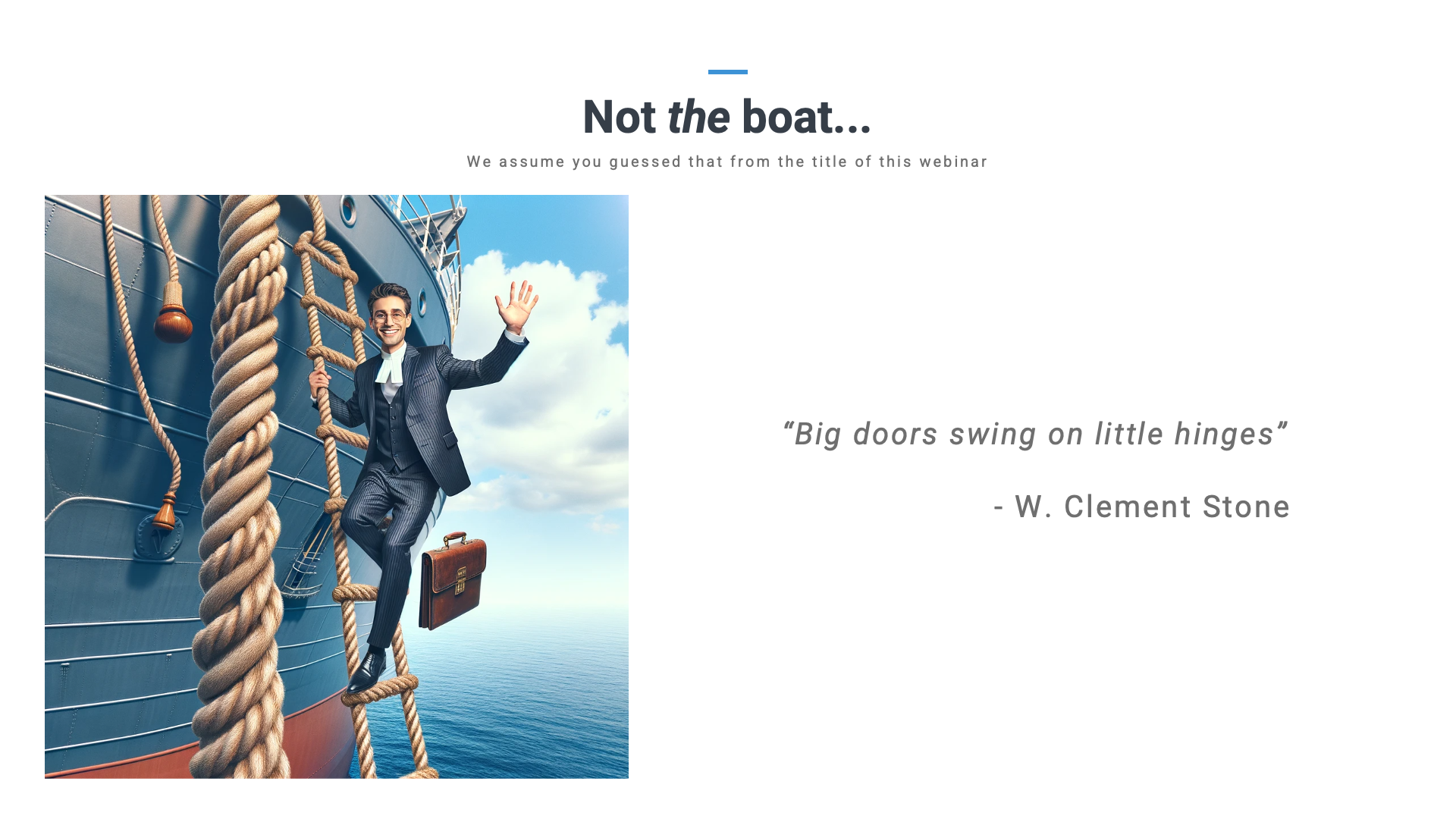
… and you’ll be happy to hear that the answer is no. Or at least, that’s there is no single “the” boat to miss.
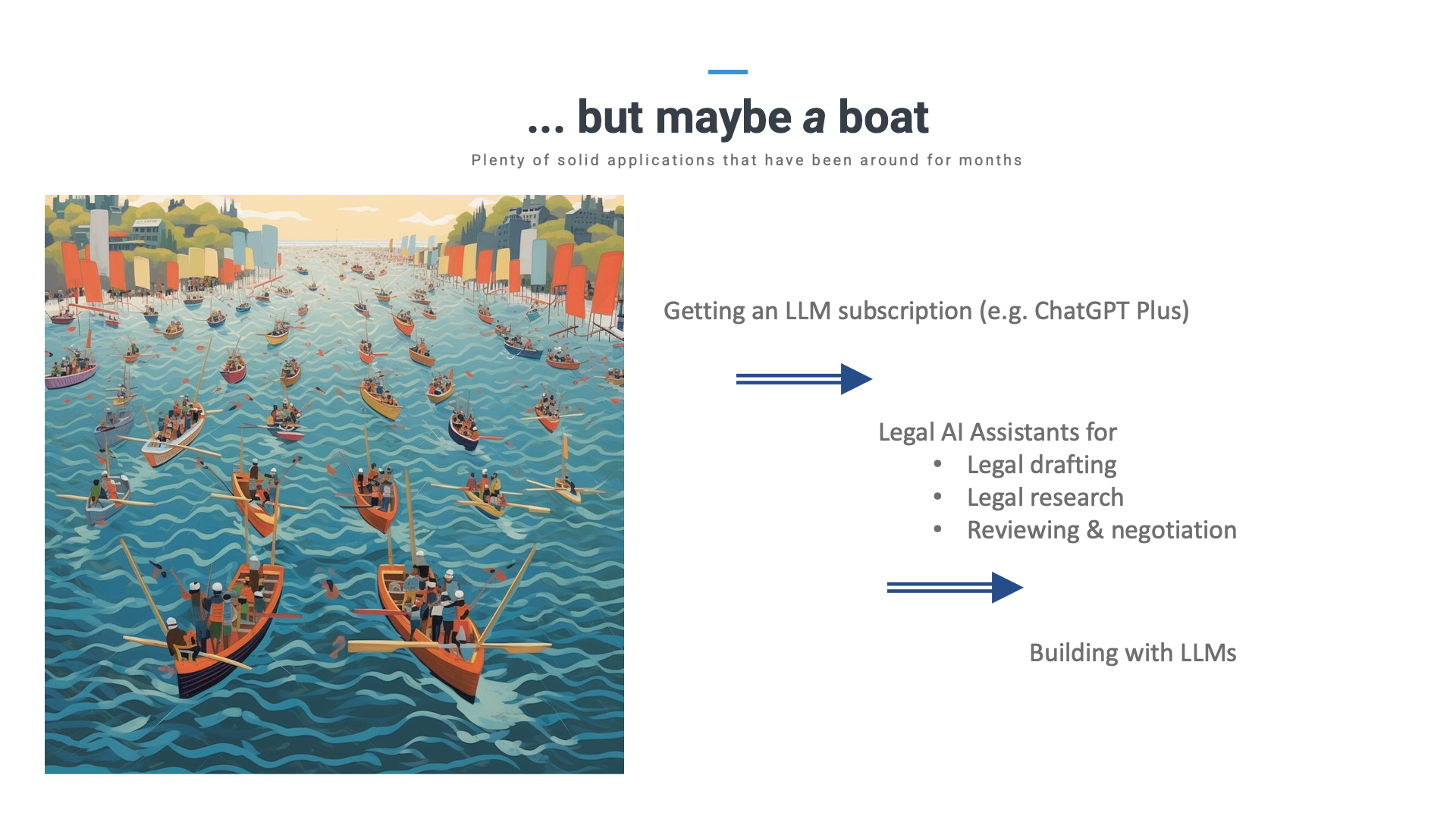
If there is anything you take away from this presentation, we hope that it is that you shouldn’t think of Generative AI as this switch you need to flick to all of a sudden be an AI-powered lawyer or anything like that.
Rather, it’s about identifying all the smaller applications that already exist. And by applications, we mean the ways in which you currently do your work that could benefit from an efficiency boost, specifically for drafting ,research, reviewing as we discussed earlier.
You can start quickly and easily here with just a simple subscription to one of the off-the-shelf models. The normal ChatGPT is free and ChatGPT Plus (which gives you access to GPT-4) costs 20 USD per user per month. Our own Legal AI Assistant ClauseBuddy also starts at 20 EUR/user/month for a single module.
In a more advanced stage, you can consider building with LLMs yourself, although this obviously requires a little more resources and expertise
LLMs are here to stay, so experiment around! Give smaller use cases a try, and be mindful of the limitations so that you apply them where they do their best work.